Bias Overview
We all have them! The goal is not to try to be free from bias, but to acknowledge them, make them explicit and find ways of minimizing their impact on processes of data collection and interpretation. Both the user (“participants”) and the user researcher (“researcher”) have biases.
Confirmation Bias
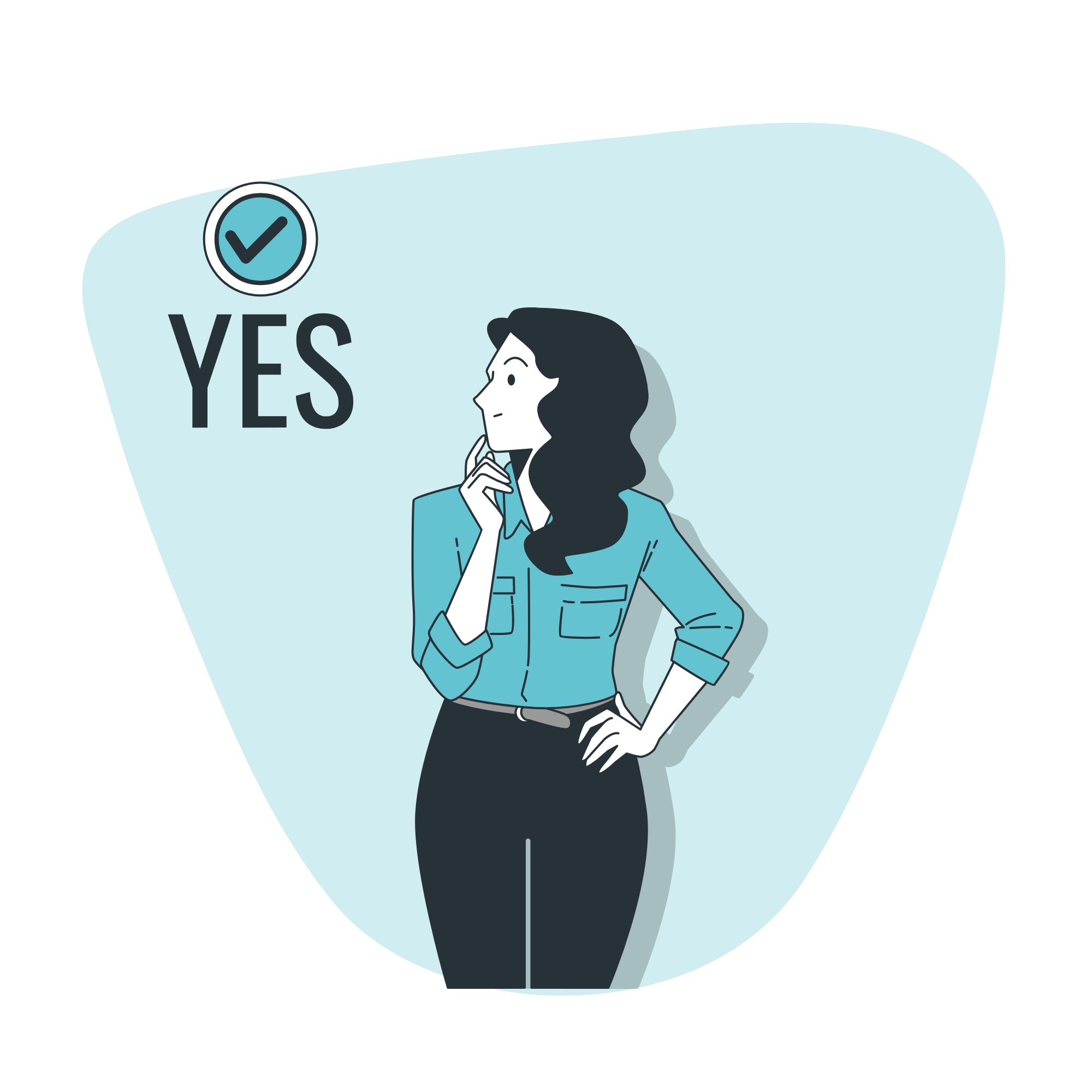
Image by Storyset from Freepik
Who is concerned?
Researchers
Concerned Methods
Data Aggregation Methods
Data Collection Methods
Effect
People tend to give more weight to evidence that confirms their assumptions and to discount data and opinions that don’t support those assumptions.
Counter Measures
- Be explicit about the metrics you are going to use to analyze user behavior (i.e., ideal time-on-task, expected bounce rate, etc.), and how will you categorize user behavior quantitatively (i.e., what percentage of test participants should successfully complete the flow)
- Be critical about positive responses and check/recheck in several different ways: Why did the user take that action? Was it because they did not have other options? Did they like the process? How many times in the last week did they do it? Can they show any evidence? Is there a possibility they might just be wanting to please you.
- Research rather than validate: start with open mind, test hypothesis and assumptions). Seek to uncover things you didn’t know beforehand, not to confirm your expectations
- Involve fresh eyes in research planning and analysis – e.g. a colleague not involved
False-consensus Bias
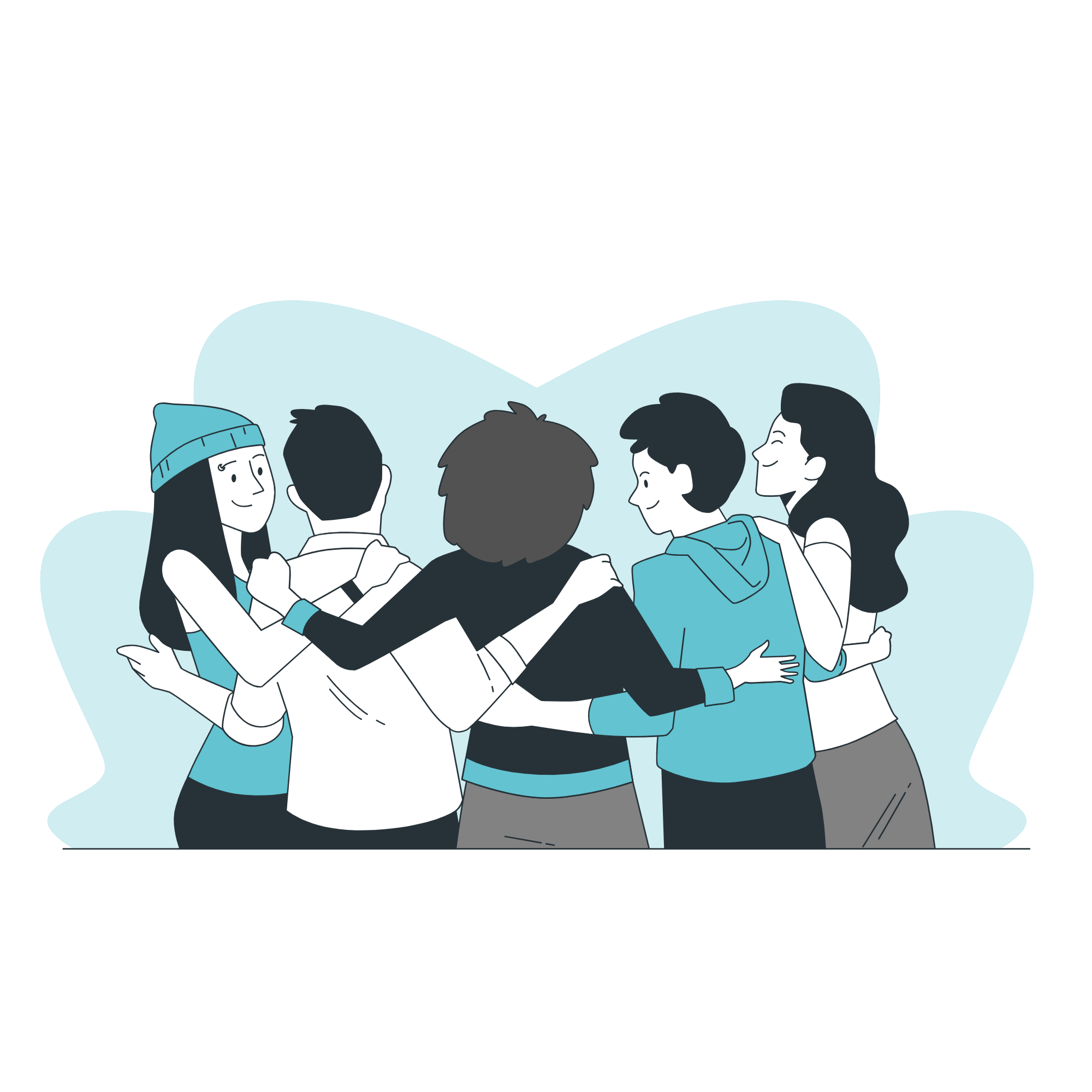
Image by Storyset from Freepik
Who is concerned?
Researchers
Concerned Methods
Data Aggregation Methods
Effect
False consensus is the assumption that other people will think the same way as you. May lead to assuming own logic chains and preferences guide users as well.
Counter Measures
- Be self aware of how you look at data – define assumptions
- Be explicit about the metrics you are going to use to analyze user behavior (i.e., ideal time-on-task, expected bounce rate, etc.), and how will you categorize user behavior quantitatively (i.e., what percentage of test participants should successfully complete the flow)
The Recency Effect
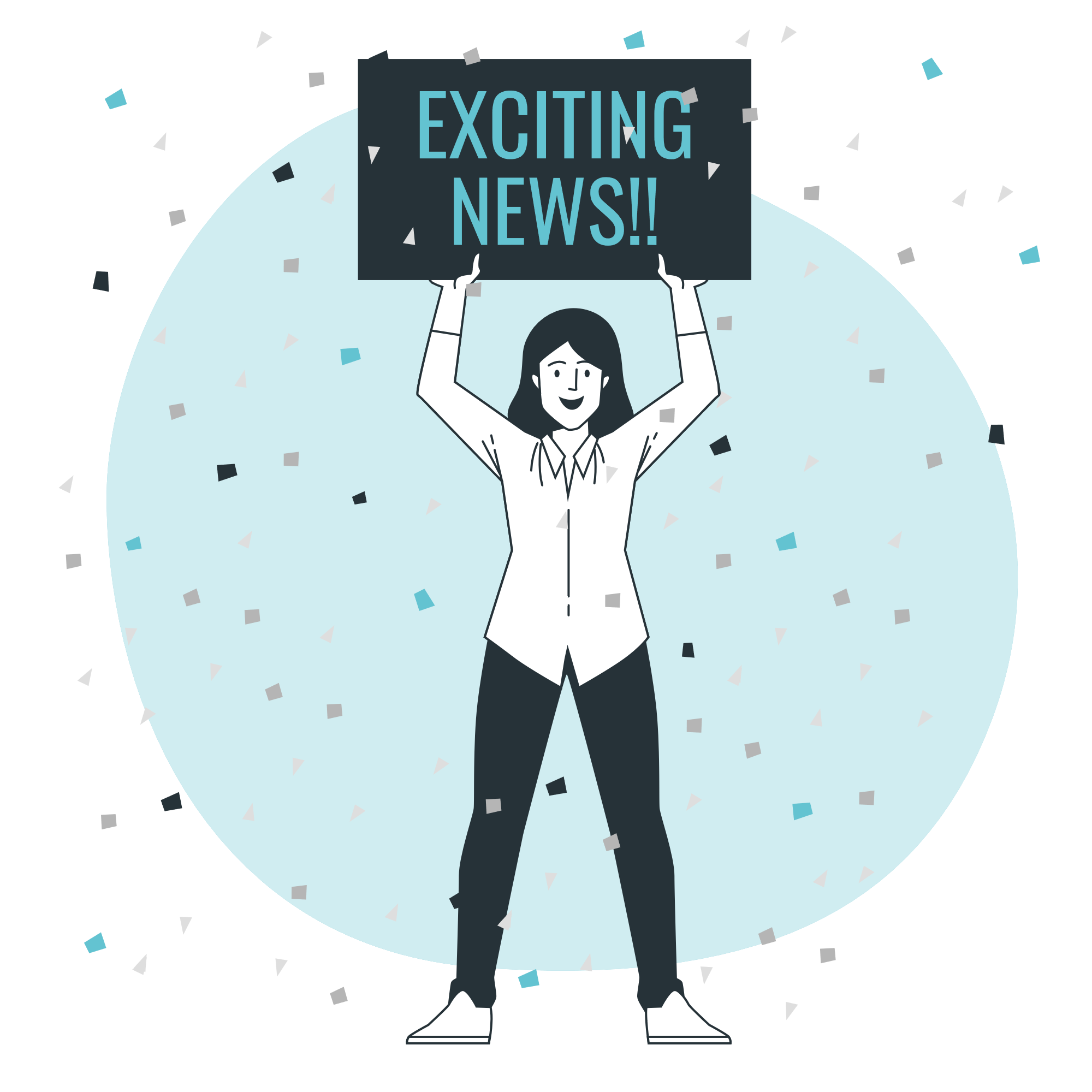
Image by Storyset from Freepik
Who is concerned?
Researchers
Concerned Methods
Usability Testing Methods
Effect
People tend to give more weight to their most recent experiences. They form new opinions biased towards the latest news, e.g. by focusing only on the problems found in the latest usability session
Counter Measures
Be explicit about the metrics you are going to use to analyze user behavior (i.e., ideal time-on-task, expected bounce rate, etc.), and how will you categorize user behavior quantitatively (i.e., what percentage of test participants should successfully complete the flow)
Anchoring Bias
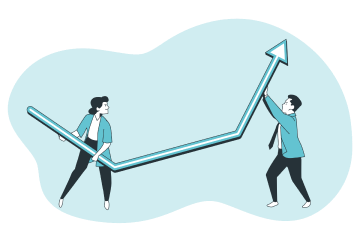
Image by Storyset from Freepik
Who is concerned?
Researchers
Concerned Methods
Usability Testing Methods
Effect
When people make decisions, they tend to rely too heavily on one piece of information or a trait that already exists. A famous example is from Henry Ford: “If I had asked people what they wanted, they would have said faster horses.”
Counter Measures
- Insights you gain before, during, and after user research should have equal weight.
- Be self aware of how you look at data – define assumptions and criteria for actionable insights
The Peak-End Rule or Serial Position Effect
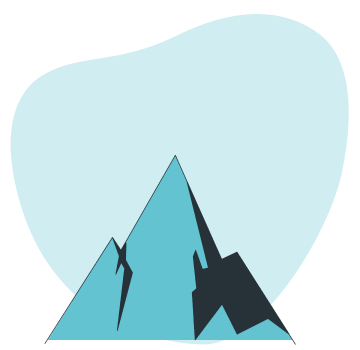
Image by Storyset from Freepik
Who is concerned?
Researchers
Participants
Concerned Methods
Methods looking for people’s opinions and perceptions (attitudinal data collection)
Effect
People tend to judge an experience more on how they felt at its most intense point (snapshot one) and its end (snapshot two) rather than based on the total sum or average of every moment of the experience. The remembered value of snapshots dominates the actual value of an experience (positive or negative). For researchers, they might tend to overemphasize results from their first and last interview (e.g. in a row of 5-10).
Counter Measures
- Try to serve and take in smaller chunks of information (limited number of interviews per day)
- Randomize information arrangements.
Social Desirability / Friendliness Bias
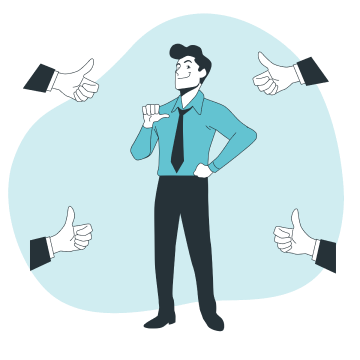
Image by Storyset from Freepik
Who is concerned?
Researchers
Participants
Concerned Methods
Data Collection Methods
Effect
People tend to make more “socially acceptable” decisions when they are around other people. Same holds true for interviews, people want to make you feel good and will answer what they think you find pleasant and acceptable.
Counter Measures
- Don’t talk too much. Let your test participant speak instead. Listen to them and observe their reactions. Ask clarifying questions such as “Why do you think so?” to let interviewees express their ideas.
- Watch your body language. Good user researchers are neutral in their reactions.
- Remember that you don’t have to become friends with users, your job is to understand their thinking, even if that involves awkward silences or small disagreements
Clustering Illusion
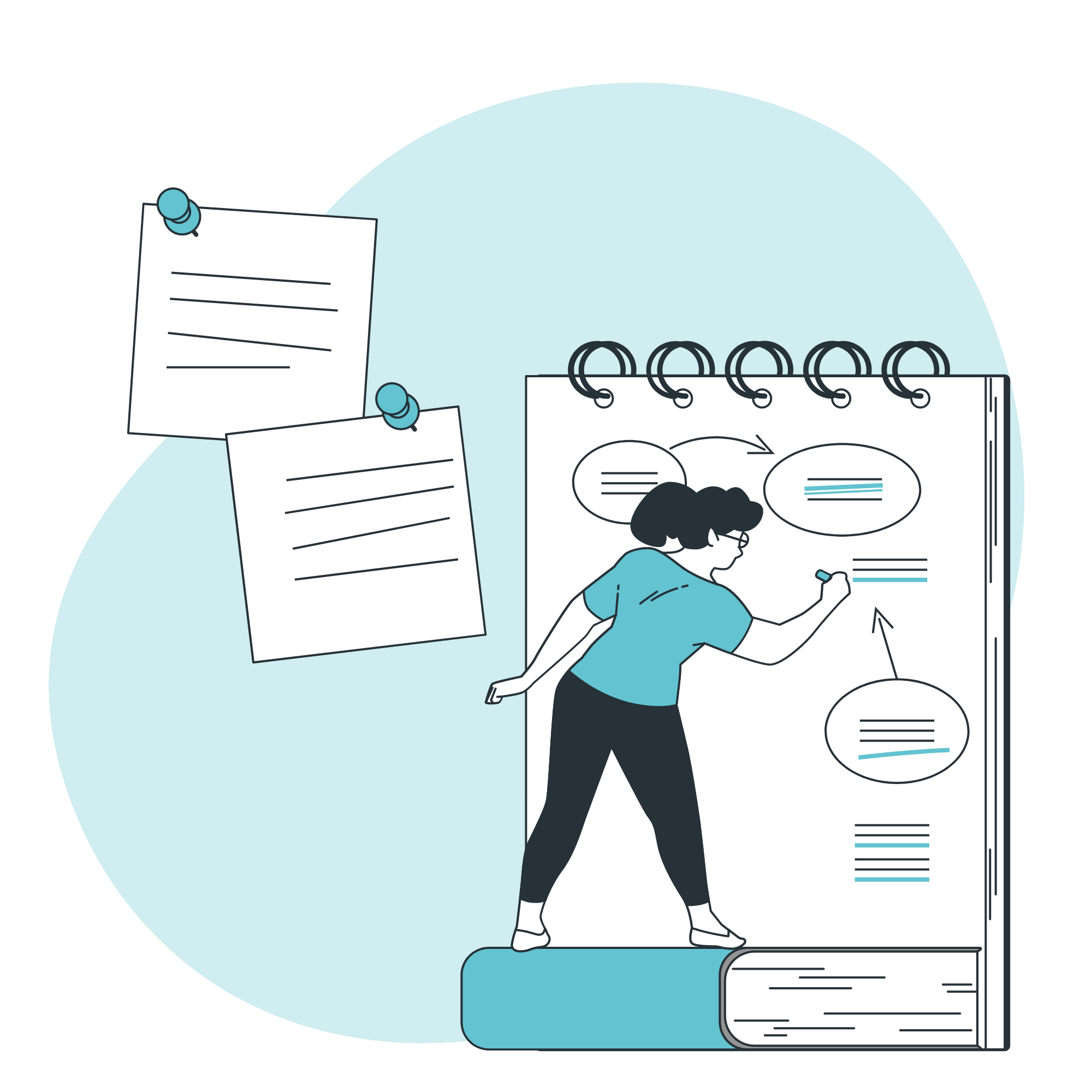
Image by Storyset from Freepik
Who is concerned?
Researchers
Concerned Methods
Data Aggregation Methods (especially qualitative methods with smaller samples)
Effect
Many UX beginners make false clusters when they analyze data and tend to see patterns even when there aren’t any. A small sample size makes it harder to understand whether the user behavior is typical for larger user segments, increasing the risk of an incorrect assumption.
Counter Measures
- Check the adequacy of your sample size & representativity
- Triangulate methods to match data-driven insights from large sample sizes with deeper insights from qualitative research
Hindsight Bias
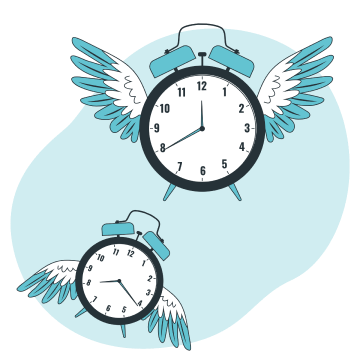
Image by Storyset from Freepik
Who is concerned?
Participants
Concerned Methods
Data Collection Methods
Effect
The hindsight bias refers to people attempting to subconsciously filter memories of past events through the lens of present knowledge.
Counter Measures
- Observe and watch your users in action to collect a broader range of data points in addition to self-reported data.
- Try to time your interviews around the events in the users’ lives that you are interested in. (e.g. interview on harvesting practices during harvest season)
Sunk Cost Trap
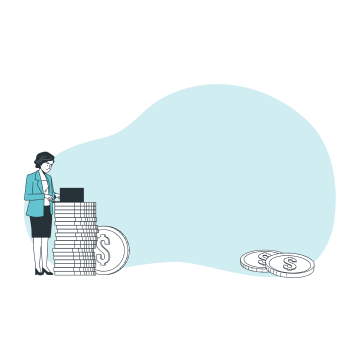
Image by Storyset from Freepik
Who is concerned?
Researchers
Concerned Methods
Data Aggregation Methods
Effect
It is very difficult for people to give up something when some resources have already been invested in it. Leads to confirmation bias.
Counter Measures
- Start testing and feedbacking as early as possible – balance efforts and rewards. This means breaking down the research into smaller chunks and having go/no-go decisions after each of those chunks
- Identify assumptions to be tested and define clear ways of looking at the data you will be collecting
Implicit Bias/Stereotyping
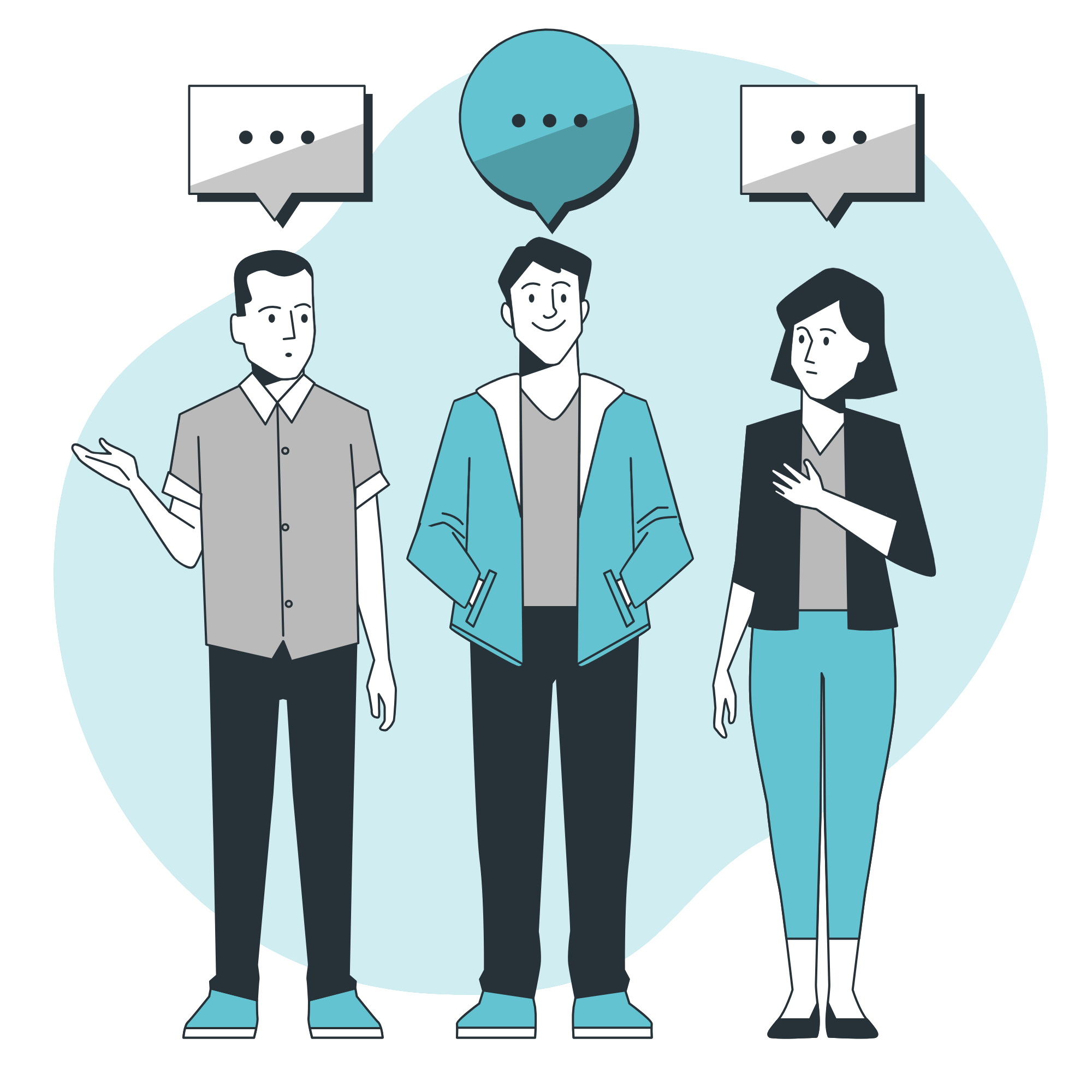
Image by Storyset from Freepik
Who is concerned?
Researchers
Participants
Concerned Methods
Data Collection Methods
Data Aggregation Methods
Effect
We associate our attitudes and stereotypes to people without our conscious knowledge. Our observations and interpretations of data can be steered by that and produce biased results.
Counter Measures
- Write down preconceived notions about the respondents of any test/interview
- Don’t over-research interviewees in order to be able to be present in the meetings
Attribution Error
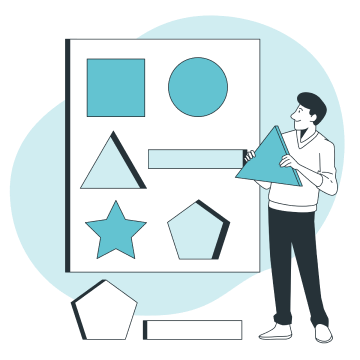
Image by Storyset from Freepik
Who is concerned?
Researchers
Participants
Concerned Methods
Data Collection Methods
Effect
Tendency of people to overemphasize personal characteristics and ignore situational factors in judging others’ (or their own) behavior. E.g. user thinks they made a mistake – good user experience doesn’t “make you think” but helps you getting things done!
Counter Measures
- Pay attention to users talking about themselves making errors. This might be an entry point to improve the experience
- Complement interviews with observation, never push blame on the user side or guide users to correct usage
Essential Reading
Sample Size Definition